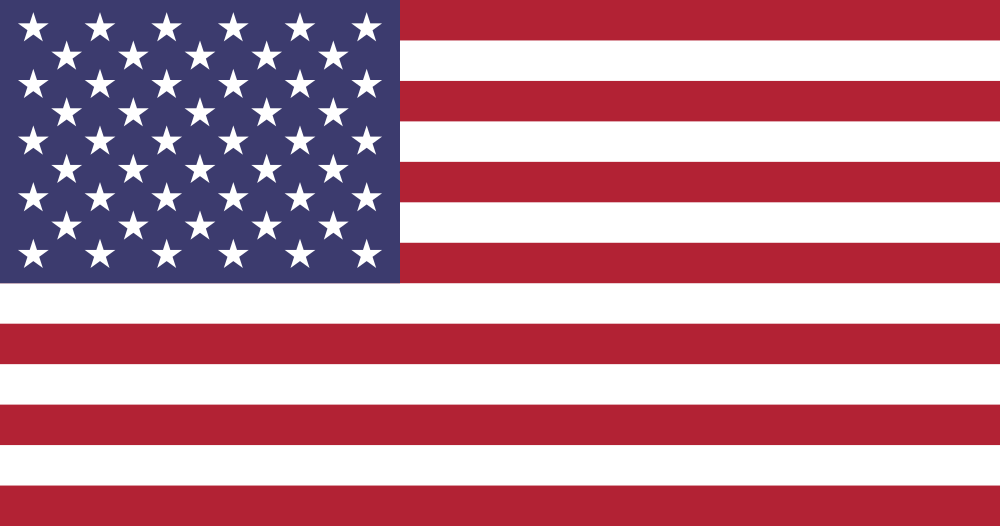
USA
HRDAG’s analysis and expertise continues to deepen the national conversation about police violence and criminal legal reform in the United States. In 2015 we began by considering undocumented victims of police violence, relying on the same methodological approach we’ve tested internationally for decades. Shortly after, we examined “predictive policing” software, and demonstrated the ways that racial bias is baked into the algorithms. Most recently we have returned to considering the challenges of documenting police violence. Our ongoing work involves collaboration with several US-based partners, including the ACLU, the Innocence Project, the Invisible Institute, Kilometro Cero, and more, to document police violence and other misconduct.