Using Machine Learning to Help Human Rights Investigators Sift Massive Datasets
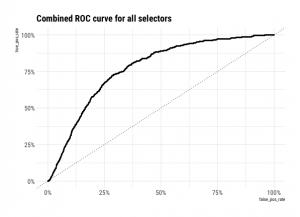
Important evidence about serious human rights abuses may be hidden among a much larger volume of mundane material within the communications and records of the perpetrators. Analysts can’t always review all available records because there may be too much of it. It turns out that we can use machine learning to identify the most relevant materials for the analysts to review as they investigate and build a case.
In this post, we describe how we built a model to search hundreds of thousands of text messages from the phones of the perpetrators of a human rights crime. These are short messages, often containing slang, variant spellings, and other unconventional language, most of which are irrelevant to the crime we are investigating. Our solution required modeling for different categories of interest to analysts, and dealing with high levels of sparsity in both labels and features.
Read the full post, co-authored by Patrick Ball and Tarak Shah, here: Indexing selectors from a collection of chat messages.